Scalable Audio Features for Clustering and Classification with Privacy Constraints
Ubiquitous computing and the Internet of Things will lead to a world where sensors are everywhere and huge amounts of sensor data are collected, processed, and stored. Without doubt, the aims of these sensor networks such as the support of elderly people (AAL), increasing security of public spaces, or improving the energy efficiency of buildings are beneficial per se and beyond question. However, many of these sensor networks are conceived to observe human activity in rather private settings, and as a consequence, significant privacy issues emerge. Among the available sensors, video and acoustic sensors are probably perceived as the most intrusive and disturbing. It is clearly foreseeable that the ubiquitous presence of acoustic sensors would have a strong effect on conversations, thoughts, and human behavior in general and, as a consequence, would not find much acceptance.
The objective of this research project is to explore the balance between data utility and data privacy in the context of acoustic sensor networks. We conjecture that audio analysis and classification tasks can be performed on the basis of privacy-preserving audio features which are aggregated to varying degrees across the dimensions of time, frequency, and space. Therefore, we will use features which are scalable across these dimensions and which will allow the control of the balance between their performance in clustering and classification tasks and privacy. To this end, we will consider a selection of clustering and classification experiments and a corresponding taxonomy of privacy levels. We will analyse the privacy of feature sets at various levels of detail in terms of information theoretic measures and juxtapose these objective measures to the feature utility in practical applications.
Contact
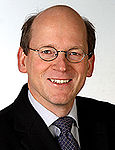
Contact
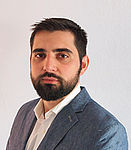